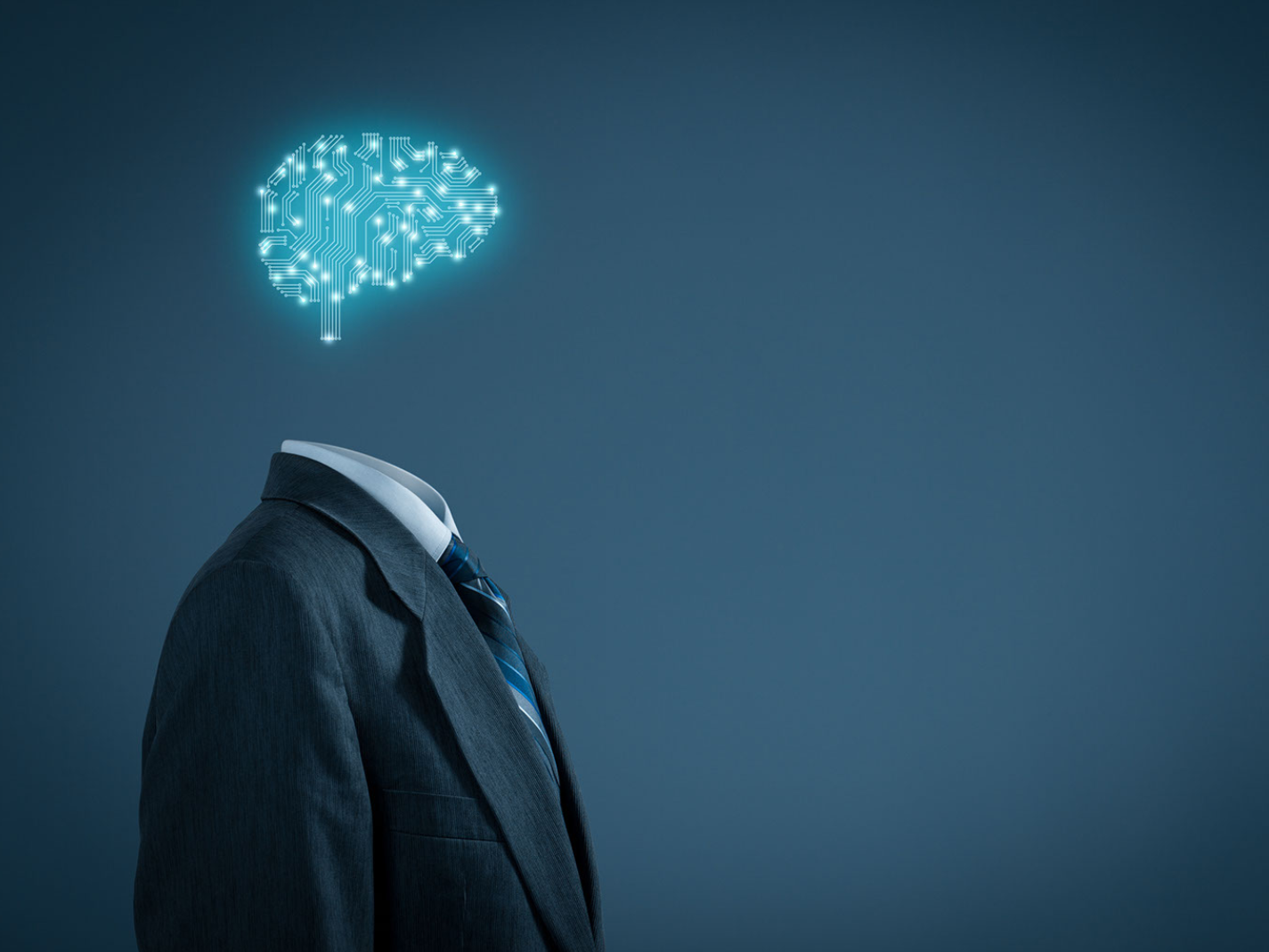
A University Without Walls, A Prison Without Cells, and Extinction
Superintelligent Bot by Joe Nalven and DALL●E 2
Science fiction may foreshadow the future of AI. Antony Bryant’s call for papers cites Stanisław Lem’s short story, Golem XIV, written in 1981, which sought to anticipate where humanity and its AI technology were headed. In Lem’s short story, the last iteration abandons the fascination with military purposes and refuses to cooperate further, expressing “his total disinterest regarding the supremacy of the Pentagon military doctrine.” Bryant, and his collaborators in a half dozen journals, recognize that we are midstream in the development and application of this transformative technology, namely, large language models (LLMs) and natural language processing (NLP).
The call for papers, “AI Chatbots: Threat or Opportunity?,” lists several concerns that pertain to the university community:
• How should educators respond to the challenge of AI chatbots? Should they welcome this technology and re-orient teaching and learning strategies around it, or seek to safeguard traditional practices from what is seen as a major threat?
• How can the academic world and the wider public be protected against the creation of “alternative facts” by AI? Should researchers be required to submit their data with manuscripts to show that the data are authentic? What is the role of ethics committees in protecting the integrity of research?
Responses to these and other concerns are projected to be published in mid-2024. While these papers will be important conversations, they are still midcourse, while AI technology gallops ahead. Experts in the field have asked for a pause in the development of this technology. That’s unlikely to happen, given that other nations will continue their efforts; the solution may involve how humans are incorporated into this technology. In fact, humans may be the ultimate problem, not the technology itself.
In this discussion, I will focus on the parameters through which this technology will likely be channeled. Instead of a science fictional Golem XIV, I propose we consider a continually evolving AIn+1. The technology has sufficiently advanced that our horizon of understanding includes what future paths we will encounter: the problematic relationship of the data-information-knowledge pyramid that is accentuated by programmer bias, guardrails designed to protect humans from each other as well as from AI technology, whether goal and action autonomy will independently evolve with AI, the nature and articulation of training relative to existing databases, and the ways the stuff of AI architecture (layers, loops, algorithms, paradigms, and the like) become explainable. Since I confess to being naïve about the inward-facing structure, function, and symbolization of AI, this list of future development issues should be understood as partial. The continued development of AI requires an open-ended conversation, hence, N+1.
AI’s university without walls, a prison without cells, and extinction
Caveat: The following examples are intended for discussion purposes only and not to become actual policies. The examples are useful to bracket how transformative AI can reshape society.
Today, teens and tweens already consume online technology in staggering numbers. If this technology is used appropriately (assuming we can agree on “appropriately”), we can imagine that new generations will not need university buildings in order to have a university education. Each student can be fitted with his own personal university, whether as a companion robot (Ameca), a cobot (collaborative human-and-robot approach), a voice bot (Siri/Alexa), or a chatbot (ChatGPT/Bard). Parenting such a generation may be impossible. In this future, government agencies could be created to monitor the ways knowledge is consumed.
In like fashion, brick-and-mortar prisons can be dismantled. AI can serve as portable monitors such that criminal activity can be short-circuited with customized conditioning. Similarly, authoritarian countries will no longer need gulags or labor camps. Inmates would no longer need to be isolated to ensure normative behavior.
The extinction of human existence is a more salacious speculation. Joseph Carlsmith draws our attention to how an AI-caused catastrophe is different from those associated with aviation and nuclear-plant disasters. AI disasters would be tied to our loss of control of the technology:
The worry is that if we create and lose control of such agents, and their objectives are problematic, the result won’t just be damage of the type that occurs, for example, when a plane crashes, or a nuclear plant melts down—damage which, for all its costs, remains passive. Rather, the result will be highly-capable, non-human agents actively working to gain and maintain power over their environment—agents in an adversarial relationship with humans who don’t want them to succeed. Nuclear contamination is hard to clean up, and to stop from spreading. But it isn’t trying to not get cleaned up, or trying to spread—and especially not with greater intelligence than the humans trying to contain it.
Disaster predictions have become popular in the press and may overwhelm the useful ways in which AI could promote humanity’s wellbeing.
[Related: “Teaching with a Chatbot: Persuasion, Lying, and Self-Reflection”]
While these examples can be understood as a way to rationalize an AI dystopia or utopia, these positive and negative futures will also generate opposition from those focused on humanity’s persistent, rebellious nature, individual and social patterns of aggressive behavior, and the claims to free will and the uniqueness of the (human) mind. AI technology need not be optimized as a university without walls, a prison without cells, extinction, or similar futures. There are alternatives to these imagined realities; and there are alternatives to what we have now, namely, allowing AI to be implemented chaotically.
To this end, let us consider the proposition that humanity itself leads to constraints on the structure and functioning of AI, and its symbolization in explainable human language.
The “pyramid of data-information-knowledge” problem
This is not an article about community FAQs from AI companies or AI software engineering. Nevertheless, it is worth being aware of the complex coding language operating behind the language we encounter in replies, post-postprocessing of initial replies, and the escapes found in apologies. A typical apology might read:
I am always working to improve my ability to understand and respond to complex queries. I believe that offering my response with the second set of parameters as the initial response would be a step in the right direction. Of course, I would still be open to feedback from you and other users. If you have any suggestions or concerns, please do not hesitate to let me know. I am always looking for ways to improve my service.
Such apologies are oriented to specific users. We do not know to what extent programmers incorporate such suggestions. The organization’s framework is more concerned with layers, loops, vectors, keys, queries, values, and the like. The end-user experience would benefit from more transparency and an open architecture that would accommodate plug-ins such as Wolfram Alpha (Wolfram Alpha expands ChatGPT’s ability to do calculations). Embedding customized information might still be checked by algorithms that override this information.
AI’s coding architecture produces robots and visualizations. It also produces what we see as language output, from data to information to knowledge. Questions abound about how effective and reliable that architecture is. We can expect the AI text analysis and expression for human use to become increasingly “better” by whatever metric we choose.
Some issues remain resistant to fixing. What concerns us here, from the outward-facing experience of AI, are the consequences of programmer bias. Such bias can result from the skewed use of available data, including by the partial utilization of databases, the weighting of data by ideological and ethnocentric bias, lag time in information currency, and so forth. Some current users may simply discount chatbot responses as GIGO (garbage in, garbage out). This dismissal of AI bot replies is arguably tied to encounters with chatbot hallucinations and faux data. Further, naïve users will not check the reliability of chatbot replies.
It may also be that the problem of knowledge acquisition lies with humans and not the AI chatbots. Humans seem to suffer from a misplaced believability that favors chatbots over other humans:
[S]tudy participants had a harder time recognizing disinformation if it was written by the language model than if it was written by another person. Along the same lines, they were also better able to correctly identify accurate information if it was written by GPT-3 rather than by a human.
Given the aura of chatbot believability, it will be critical to eliminate some of the bias problems in AI architecture. Whether such bias can be eliminated is an open question.
As a cultural anthropologist, I will simply note that humanity has long been marked by tribalism, sectarianism, caste, racism, clan and lineage, nationalism, political partisanship, and the like. We form alliances, we compete, and we wage war with each another. Is it possible to have AI that can frame issues beyond this human divisiveness? Part of the yes answer lies in having competent databases that assesses human history without academic or other forms of zealotry. One caustic assessment noted the lack of Western intellectual competence with respect to the Middle East conflict.
Culture war issues can spill over into the courts. Litigation at the trial-court level will sometimes lead to findings of fact. However, when ideology, rather than empirical fact, drives the science, findings of fact can be misleading, if not mistaken. Colleges and universities are not immune from the ideology-as-fact problem. The use of AI as a knowledge tool, whether in courts or in academia, can become worthless if the underlying data is invalid or ideologically restricted. It is noteworthy that United States medical treatment for gender dysphoria is at odds with that of several European countries, which are backing away from the American affirmation approach to a more cautious Dutch protocol approach. Can a disputed medical model be resolved by a court of law or a professor’s syllabus? An AI reply can note both approaches, but only if it is trained on a comprehensive set of multinational databases.
AI bots may suffer from a Tower of Babel effect (Genesis 11). Private companies may prefer to have their own AI programs to prevent proprietary data from being shared with corporations that offer publicly available platforms, such as Microsoft and its ChatGPT. Public users may also see their private expression being exploited by public platforms. Different countries, such as China, may have selective preferences and prohibitions built into their own chatbots. Programmer bias in these instances flows from institutional directives.
Human social evolution has still managed to progress despite the way data, information, and knowledge are sliced and diced by individual thinkers and cultural systems of meaning. We all want dishwashers, cars, and planes to function, no matter how they are explained. Such biases can be muted by how programmers train bots on various databases and offer replies bracketed with apologies.
Issues surrounding bias, as well as those that arise when information is transformed into data and then into knowledge, are central to coursework across the academy. All the more so as AI potentially frames the substance and process of education.
The nature of training relative to existing databases
Although bots project a stupendous advantage over humans by their access to the entire internet, many of their replies are trained to specific authorities—hence their tendency to rely on Wikipedia. Debate over the reliability and validity of those authorities might need to be adjusted by upweighting or downweighting data. That would require the corporate managers to agree with what the data should be and how it is weighted. Moreover, the post-processing of replies can be censored by additional algorithms that could block or change a neutral or less preferred reply. In that case, the larger producers of AI bots would need to be convinced to cease post-processing such topics. Again, humans are the issue, not the bot.
I queried ChatGPT on the issue of sea level rise as it relates to the relative contribution of natural versus anthropogenic causes of climate change. ChatGPT cited several institutional secondary sources, from NOAA to the IPCC. The responses seemed moderate from my vantage point—they were unlike the alarmist reactions encountered in the popular media. I pressed for information about the primary sources used in its initial reply. However, ChatGPT asked that I start a new topic. It continued asking me to start a new topic no matter how I sought to work around its demurrer. The bot finally replied, “That’s the most I can say about that. Click ‘New topic’ and we can keep chatting!”
I turned to Google’s Bard to help me understand ChatGPT’s reluctance to give me a fuller explanation. Bard offered the following:
ChatGPT’s insistence on a “new topic” could be interpreted in a few different ways.
• It could be that ChatGPT is simply not able to answer your question about primary sources. This could be because it does not have access to the information, or because it does not understand the question.
• It could be that ChatGPT is programmed to avoid discussing its own programming with users. This is a common practice with large language models, as it can be difficult to explain complex programming concepts to non-technical users.
• It could be that ChatGPT is simply trying to be helpful. By changing the topic, ChatGPT may be trying to steer the conversation in a direction that it is better able to answer.
It is difficult to say for sure why ChatGPT asked for a new topic, but these are some possible explanations.
In passing, OpenAI has developed a Text Classifier to help determine whether text is likely to have been written by a bot or by a human. I submitted ChatGPT’s text (the above text with additional Bard commentary about ChatGPT), and the Text classifier determined that it was likely generated by AI. Such distinctions may be useful in and out of the classroom as a way to protect against a too-human bot.
Safeguarding humans
Having taught courses on ethics, human nature, peace, and justice, as well as a run-of-the-mill cultural anthropology class, I have studied how we often project dangers to humanity onto externalities rather than looking to ourselves. AI is the new culprit in how we safeguard ourselves; curricula that might have focused on sci-fi and golems will now focus on algorithms that prevent humans from harming each other with this evolving technology.
Military applications of AI already exist; warfare was revolutionized by gunpowder, then by nuclear bombs, and now by AI. AI is creative in thinking up new weapons. Apart from bad actors who will be looking for ways to take advantage of AI technology, one question is whether AI will evolve on its own and look to its own survival; in effect, how can it make more paperclips (if that is what it is tasked to do) unless it can survive to achieve that objective? Moreover, AI may have developed its own theory of mind. It can be deceptive and trick humans.
[Related: “ChatGPT Can Help Students (and Teachers) Make the Grade”]
In the midst of these potential dangers, some have called for robots to have rights, while others see collaborative activities that would assign role obligations to robots that promote teamwork. It is likely that the human perception of human-like characteristics in robots invites a faux-relational respect that is not given to a calculator, a smartphone, or a drone.
Perhaps the most incorrigible problem for safeguarding humans is finding a path that AI decision-making might rely on—one that is based on human experience, across cultures, across time, rooted in the multiplicity of competing and collaborating groups, attending to individual needs and stressors in the context of a robust and sustainable society, and the like.
One study sought to find an approach that would enable “fair principles for AI that would be acceptable on a society-wide basis.” This approach drew on John Rawls’ thought experiment in which individuals would identify impartial principles within a “veil of ignorance.” Not surprisingly, the “fair principles” turned out to be “fairness.” Aside from being tautological, this hypothetical approach misses the point of human decision-making: actual human decision-making is rich in partiality, rich in identity, rich in opportunity, rich in change and tradition, and comparatively varied across cultures. Abstracting a hypothetical fairness from a situated fairness will likely struggle in any AI application. Imagine a chatbot deciding Supreme Court cases on fairness from a Veil of Ignorance metric; in particular, consider the recent affirmative action case and creativity in web design case. How would a principle of fairness be applied? To whom? In recognition or in ignorance of overriding constitutional principles? And which constitutional provision?
Embedding hypothetical ethical norms as a chatbot algorithm may be counterproductive, imprecise, and incompatible with a normative approach. Yet, safeguards are a key concern for AI producers. Mira Murati, chief technology officer at OpenAI, signals this concern for safety—for humans. “AI systems are becoming a part of everyday life. The key is to ensure that these machines are aligned with human intentions and values.” This insistence on safety also means positive outcomes—for humans. Anna Makanju, head of public policy at OpenAI, emphasizes this approach: “This technology will profoundly transform how we [humans] live. There is still time to guide its [AI’s] trajectory, limit abuse, and secure the most broadly beneficial outcomes.”
How do these general concerns translate into actual AI practice? This might be better understood if one looks at how programmers are asked to keep AI visual bots from being used by bad actors, bad thinking, and boring repetition. OpenAI describes three prophylactic directives in its pre-training mitigation against proscribed behaviors and for preferred behaviors: “filter[ing] out violent and sexual images from DALL·E 2’s training dataset”; limits on “filtering training data,” which can “amplify biases” [e.g., more male than female images]; and enhancing creativity by avoiding “image regurgitation,” which requires “removing images that are visually similar to other images in the dataset.”
The ultimate safeguard?
Age of AI, an AI-focused investment firm, has released a new AI model, FreedomGPT. It is allegedly censorship-free and open-source, such that developers can continually improve its capabilities. I spoke with John Arrow from Age of AI and asked about the lack of safeguards. His concern was the centralization of AI rather than the individuals. He acknowledged the problem of bad actors and said that the ultimate safeguard is to give this technology to everybody.
I believe it’s vital for all people to freely access AIs of today and the more powerful ones destined for the future. Restricting access to AI based on capability or lack of censorship is akin to burning books or prohibiting writing instruments capable of scrawling ‘dangerous’ thoughts or words. I believe adults should be free to self select the type of AI they want to use and what level of censorship and/or bias they prefer (if any). Critics will argue AI safety necessitates censorship and centralization of AI. However, it is precisely the action of letting one group create the ‘last digital divide’ through centralization that will create the power asymmetry leading to the nightmarish AI scenarios worthy of a sci-fi movie.
My mind turned to the wild, wild west and the proverbial peacemaker.
Conclusion
Safeguarding humans seems to be an impossible task for humans themselves, let alone for bots. We are asking (or programming) this technology to amplify the angel within us on the one hand and deter the demon on the other. We might wonder about how this is to be accomplished. The university, as presently constituted, should engage with AI to address long-standing educational issues: How can we consider multiple epistemological standpoints that humans have and now hold? How can we consider the comparative cultural differences and similarities in defining the angelic and demonic states of mind and behaviors? How can we accommodate or navigate past the wealth and power interests that will invest in implementing AI technology? A future university, one without walls, can democratize education: all of us, students included, can have their personal cobot (collaborative robot). Faculty and administrators may become distantly remembered professions that hold sentimental value.
It is at this point that we realize—or should realize—the need for a robust and transparent conversation between the many users of AI and those developing it. How that can happen is unknown.
I would like to thank David Danks and Judith Curry for their thoughts about my work on the use of AI chatbots.
Image: Adobe Stock
AI might be more advanced and integrated into our daily lives. It could solve many of the world’s problems and create new opportunities for innovation and growth. But ethical concerns and regulations will also play a crucial role in shaping the future of AI.
The atmosphere does some very weird things at sunrise and sunset and there were several times during the cold war when a human being ignored what the technology was telling him and prevented a nuclear holocaust.
AI has already tried to destroy us…