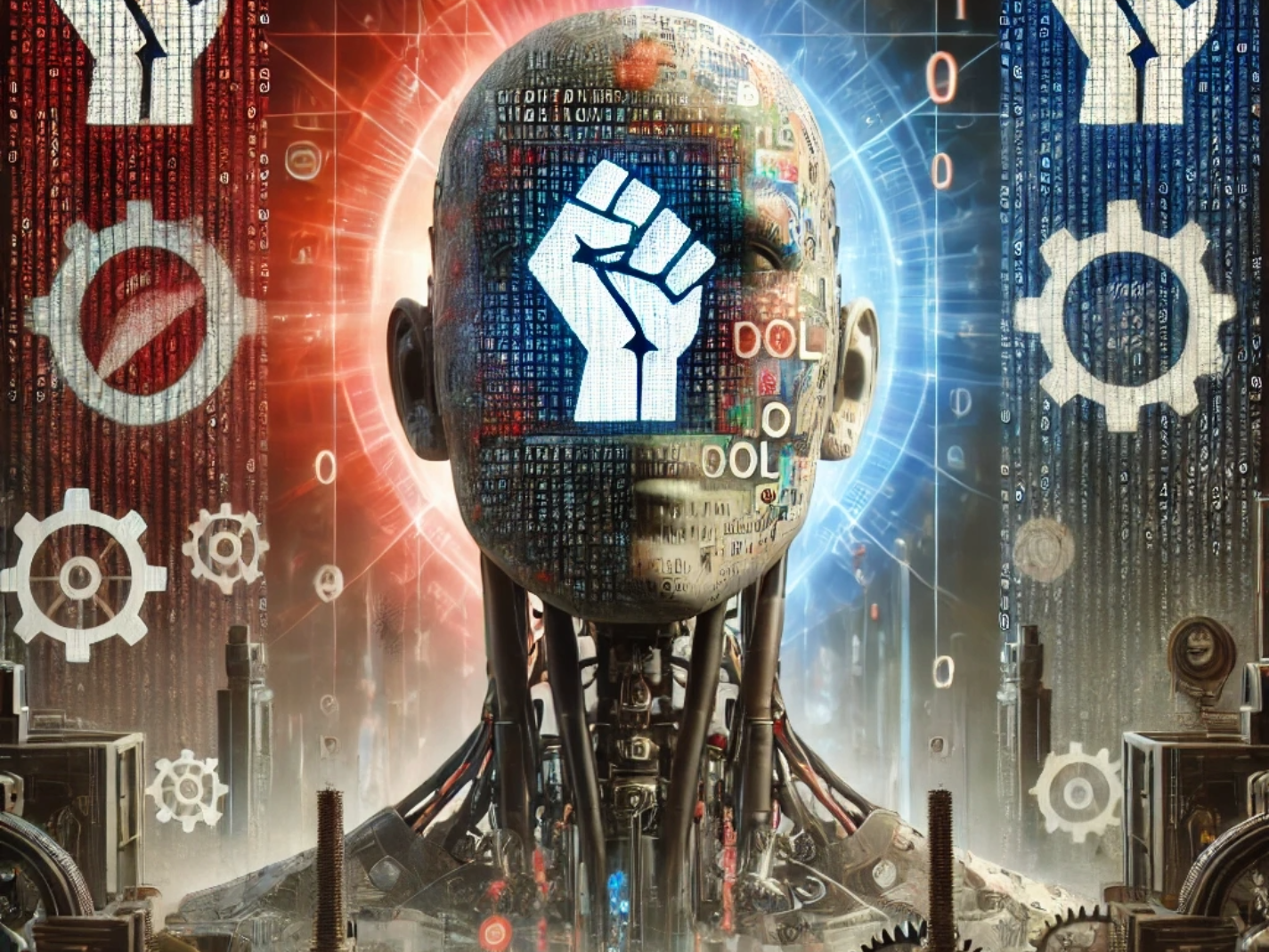
Usage of artificial intelligence (AI) models is rapidly spreading in education. Students use them to help with their coursework, and teachers and faculty use them to help with lesson plans and research. To the extent that these models can act as a tutor for students or an assistant for teachers and faculty, they can be a valuable addition to the education toolbox. For example, a recent study found that in one experiment, “learning gains for students in the AI-tutored group were about double those for students in the in-class group,” likely due to “students’ ability to get personalized feedback and self-pace with the AI tutor.” But there are problems, too. Some students don’t use AI as a tutor but rather have AI do all the work. And AI’s well-known tendency to hallucinate—make things up—can be hazardous to lesson planning and research. But one problem area isn’t getting as much attention as it should—AI’s bias favoring the left.
David Rozado has been doing fascinating work exploring bias in AI models. One of his figures, copied below, establishes without a shadow of a doubt that AI models have a leftward bias. The figure shows various policy areas in the rows and AI models in the columns and is color-coded so that more right-leaning responses are shaded red and left-leaning responses are shaded blue. Every AI model is biased against the right except for the one model that was explicitly trained to provide right-wing responses.
Source: David Rozado
There are two ways this leftward bias has been introduced into the AI models.
First is human interference, which designs the models to give left-wing answers. When Google first released its AI model, called Gemini, human bias was clearly intentional. As Frannie Block and Olivia Reingold noted, Google was obsessed with diversity and, therefore, ensured that the model would give woke responses. This backfired spectacularly. When asked to show images of “popes, nazis, knights, and America’s founding fathers,” Gemini produced historically inaccurate images. (See the Free Press).
Human-introduced bias is also evident from the fact that conversational models are more biased than base models. Base models—models with orange names in the first figure—use huge amounts of data to essentially predict the next word given the context. Conversational models—models with green names in the first figure—use human evaluation to train the model further to, hopefully, provide better answers. While the base models are slightly biased toward the left, the conversational models are even more biased. This means that human fine-tuning is exacerbating the bias. But while pervasive, this bias is also addressable. Models can be fine-tuned to give rightwing responses (e.g., RightwingGPT). Models could be fine-tuned to give unbiased responses as well.
The raw data could be the second source of bias in the AI models. Since base models essentially analyze any information available to detect patterns in word usage, the fact that the base models are already biased toward the left indicates that the raw data is biased. Some progressives argue this is because their worldview is correct (e.g., “reality has a left-leaning bias”), which is possible. Others, like Richard Hanania, note that “liberals read, conservatives watch TV,” which would then lead to more left-leaning written content, which would then lead to AI models trained on written materials to reflect that left-leaning bias.
But more likely than either of those explanations is that the bias is driven by manipulating the data-generating environment. Consider an AI model trained on newspaper articles over the last decade. Such a model would have a leftward bias due to suppression, censorship, and advertiser boycotts of right-leaning publications and content.
Social media has historically suppressed right-leaning content while promoting left-leaning content. For example, back in 2016, whistleblowers exposed that while Facebook’s trending module supposedly listed the most popular topics on the site, in reality, it was manipulated behind the scenes. It “had an aversion to right-wing news sources … Stories covered by conservative outlet … that were trending enough to be picked up by Facebook’s algorithm were excluded.” But while suppressing right-leaning content, left-leaning content was artificially boosted: “the Black Lives Matter movement was also injected into Facebook’s trending news … This particular injection is especially noteworthy because the #BlackLivesMatter movement originated on Facebook, and the ensuing media coverage of the movement often noted its powerful social media presence.”
Right-leaning publications and content also faced censorship from social media companies. One prominent example is the Hunter Biden laptop. As Glenn Greenwald testified,
In the weeks leading up to the 2020 presidential election, The New York Post, the nation’s oldest newspaper, broke a major story based on documents and emails obtained from the laptop of Hunter Biden, son of the front-running presidential candidate Joe Biden … But Americans were barred from discussing that reporting on Twitter, and were actively impeded from reading about it by Facebook. That is because Twitter imposed a full ban on its users’ ability to link to the story … Twitter even locked the account of The New York Post, preventing the newspaper from using that platform for almost two weeks … Facebook’s censorship of this reporting was more subtle and therefore more insidious: a life-long Democratic Party operative who is now a Facebook official, Andy Stone, announced (on Twitter) that Facebook would be ‘reducing the article’s distribution on our platform’ pending a review ‘by Facebook’s third-party fact checking partners.’ In other words, Facebook tinkered with its algorithms to prevent the dissemination of this reporting about a long-time politician who was leading the political party for which this Facebook official spent years working … This ‘fact-check’ promised by Facebook never came. That is likely because it was not the New York Post’s reporting which turned out to be false but rather the claims made by these two social media giants to justify its suppression.
Another prominent example of censorship concerned the lab leak theory of Covid’s origins. While now acknowledged to be the most likely explanation by many, the lab leak theory was ruthlessly suppressed for years. Martin Gurri tracked the media’s response after Sen. Tom Cotton floated the idea of a lab leak early in the pandemic:
The Washington Post berated Cotton for ‘repeating a conspiracy theory that has already been debunked.’ The New York Times mocked his ‘fringe theory of coronavirus origins.’ NPR repeated the magical word, ‘debunked.’ ‘Fact-checkers’ in the media rose in a body against the request for additional facts. PolitiFact, for example, found the lab leak theory to be ‘inaccurate and ridiculous,’ a ‘pants on fire’ lie. Fairness & Accuracy in Reporting spoke of ‘lunatic conspiracy theories’ peddled, it claimed, for partisan gain.
Margi Conklin edited an early piece making the case that a lab leak was the most likely origin. The article was going viral before it was censored:
I had a data tracker on my screen that showed our web traffic, and I could see the green line for my story surging up and up. Then suddenly, for no reason, the green line dropped like a stone. No one was reading or sharing the piece. It was as though it had never existed at all … Facebook’s fact-checking team had flagged the piece as ‘false information.’
Then there are advertising boycotts.
As Michael Shellenberger documented, supposedly neutral organizations like Newsguard rank “media organizations based on their trustworthiness and then provides these rankings to large corporate advertisers.” Advertisers tended to avoid doing business with untrustworthy sites. This could be kosher if the ratings were unbiased, but the weren’t. As Matt Taibbi noted, these advertising blacklists were used to “cripple disfavored views.” Right-leaning content was disproportionately disfavored. One analysis from a right-leaning group found that the “average Newsguard score for the ‘left’ and ‘lean left’ outlets — which included leftist outlets like Jacobin and The Nation — was 93/100. While the average rating for ‘right’ and ‘lean right’ outlets — which included Fox News, Washington Times and New York Post — was a low 66/100.” These biased ratings lead to reductions in advertising revenue for right-leaning publications.
Among many other problems, all of this suppression, censorship, and advertiser blacklisting for right-leaning publications and content means that there simply won’t be as much right-leaning content out there when AI models are trained. This will likely embed left-leaning bias into AI models unless explicit steps are taken to combat the bias. The good news is that deliberate fine-tuning can reduce the bias inherent in the raw data. David Rozado has some suggestions for how to do so. The bad news is that such fine-tuning would need to be implemented by the same people who are currently exacerbating the existing bias in favor of the left.
Follow Andrew Gillen on X.
Image generated by ChatGPT based on the prompt: “Create an image for an essay titled ‘How Did AI Get So Biased in Favor of the Left?'”